How much League of Legends data is enough data? – Esports
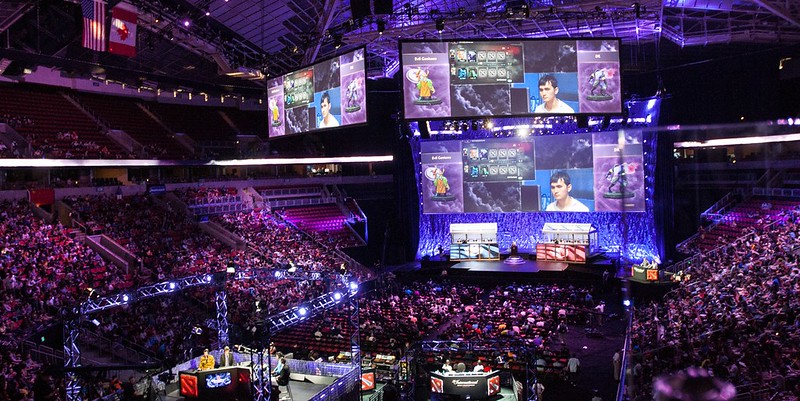
Gaweł Paprzycki of Bayes Esports delves into a critical query within the esports betting sector: how much historical data is required to formulate an optimal betting model, particularly when the inclusion of older matches ceases to provide actionable insights?
Understanding the Dynamic Landscape of League of Legends
League of Legends is a game synonymous with constant evolution. Professional players face the ongoing challenge of adapting to regular patches that revise champion capabilities, item viability, and strategic frameworks approximately every two weeks. To gain a competitive advantage, teams must decipher what strategies resonate with the current meta, ideally prior to its establishment.
The Role of Data in Betting Models
At Bayes Esports, we confront similar challenges in our betting algorithms. We leverage machine learning to forecast potential outcomes, including victories and objectives. The accuracy of our probabilistic models hinges on their ability to reflect current strengths and weaknesses within the game.
In an ideal scenario, we would train our models exclusively with the most recent data. However, given the volume of matches played in League of Legends, this simplicity is unattainable.
The Pitfalls of Over-Reliance on Historical Data
While it may be tempting to incorporate data spanning the last five years, this approach often leads to inaccuracies. Game mechanics and champion dynamics fluctuate not just with patches but also with emerging counter strategies. Thus, models must be designed to adapt to the game’s evolution while remaining resilient against abrupt shifts.
The Search for Optimal Data Relevance
Determining the relevance of two-year-old data is crucial. Where is the equilibrium between the quantity of past matches and the significance of that data to model performance? To investigate this, we conducted a focused research project that yielded promising insights.
Our methodology was straightforward. Given that League of Legends patches typically last about two weeks, we segmented our dataset into two-week intervals. We then repeatedly trained a map-winning odds model with incremental data from multiple patches, validating the accuracy of our predictions on the most recent matches excluded from the training set. This experiment was conducted with various start dates, assessing periods such as January 2022.
If the game from two years prior mirrors present conditions, we would expect model accuracy to improve with the addition of historical data. Conversely, if patches significantly alter gameplay, we would observe a decline in predictive accuracy—a factor warranting examination across different timeframes due to the non-linear nature of game modifications.
Insights Derived from Model Evaluation
To measure model efficacy, we utilized the Brier score, a recognized metric for evaluating predictive accuracy. A score closer to zero indicates higher accuracy, with a difference of at least 0.005 signifying statistical significance.
Our analysis indicates that a model predicting map outcomes, trained on approximately 5,000 matches spanning 21 patches (around 10.5 months), yielded the highest accuracy. In contrast, data from the most recent 12 patches proved insufficient for reliable forecasting. While the distinctions in accuracy were relatively subtle, they held statistical significance—these minute differences can prove critical in competitive betting against seasoned punters who meticulously track the current meta.
Patch-Specific Biases and Model Predictions
An intriguing observation involves how models developed on varying volumes of data behave differently based on patch selection. The model trained on the latest 12 patches exhibited a bias favoring the red team. In contrast, those trained on larger datasets demonstrated more balanced probabilities, suggesting inherent advantages may shift between teams in accordance with patch updates.
Optimal Data Parameters for Enhanced Predictions
Our research concludes that the most effective timeframe for data usage falls within a 6 to 12-month window, with a minimum sample size of 3,000 matches required for optimal prediction models. This intuitive conclusion reflects the reality that while patches can lead to immediate changes, player adaptation and meta-development take time. Additionally, not all patches exert equivalent influence on the competitive landscape.
Staying Ahead in the Evolving Esports Landscape
Leveraging the unique data at our disposal, it is imperative to maximize its utility. As the game evolves, Bayes Esports is committed to maintaining its leading position in the esports betting arena. Staying attuned to these shifts will ensure we continue to develop the most precise models and betting odds, ultimately enriching the betting experience for our clients.