Esports research ideas: Draft strength estimator – esports betting
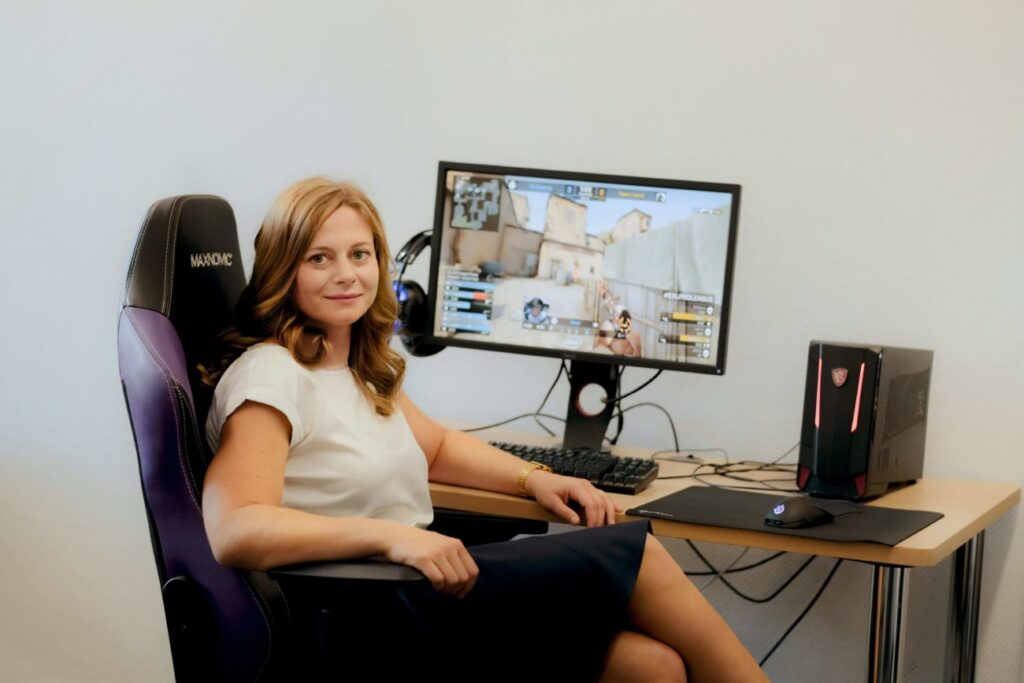
In the second installment of her insightful series, Dr. Darina Goldin of Bayes Esports delves into a transformative product idea that could significantly elevate the esports ecosystem. Although the realization of these concepts presents challenges, their benefits to the community could be immense.
At the commencement of most esports titles, players are presented with a diverse pool of characters to choose from—ranging from a dozen to over a hundred options, each embodying unique strengths and weaknesses. In team-based formats, particularly, crafting a balanced roster becomes imperative. Tactical compositions often require a blend of damage dealers, healers, and support roles to optimize team synergy and strategy.
This necessity is especially pronounced in professional matches, where team dynamics play a pivotal role in determining outcomes. The so-called ‘draft phase’ emerges as a critical juncture, influencing the trajectory of the match. While anecdotal observations provide insight into effective team compositions, empirical data supporting these claims remains scarce.
Understanding the ‘Pick and Ban’ Phase
In Multiplayer Online Battle Arena (MOBA) games, the analysis of team strategy is further complicated by the ‘pick and ban’ phase. This critical period, occurring at the outset of a match, allows teams to either select champions or ban others from a shared pool of characters. The stakes are high, as these decisions can essentially determine the success or failure of the game. The implications of this phase are manifold:
- **Champion Strength**: Is a particular champion currently overpowered, giving one team a distinct advantage?
- **Preference and Availability**: Are teams able to secure champions that align with their strategic preferences?
- **Counter Strategies**: Are there champions or combinations that effectively counter opponents’ selections?
- **Timing Dynamics**: Have teams chosen champions that are strong in the early game versus those that gain power later?
- **Blocking Key Picks**: Is one team successfully restricting access to a champion that the rival team covets?
Some champions are consistently sought after due to their superior performance. Others are situational picks crafted to outmaneuver specific opponents, while some selections are compelled by the opposing team banning desired heroes. Analysts have the capability to assign quantitative values to each pick or ban, providing evidence-based recommendations.
As the draft phase unfolds, it is essential to determine optimal strategies for the first pick. Upon selection, our methodology shifts to assess the most advantageous options for the opposing team. Depending on the dynamics, we may discover a singular standout candidate, or a spectrum of viable alternatives, which is essential for data scientists operating within esports teams.
At Bayes Esports, our ambition extends beyond merely suggesting optimal picks. We aim to conduct thorough analyses of existing compositions. Following each selection, reevaluating the win probabilities for both teams becomes crucial—especially in the context of betting.
Given the weight of the draft on a team’s winning odds, this consideration should also permeate betting markets. A failure to secure preferred champions ought to correlate with diminished winning prospects, influencing odds accordingly.
Ideally, this evaluation framework can be customized and refined, learning from historical match data. Adaptive strategies are vital, particularly post-patch, as changes in game mechanics can upend champion valuations and disrupt prevailing meta-games.
Seeking Innovative Solutions
While this challenge appears straightforward—leveraging historic data to create predictive win statistics—the underlying mathematics quickly complicate matters. With 154 champions in League of Legends and 120 in Dota 2, each professional match sees ten characters picked and another ten banned, resulting in trillions of potential team combinations. Achieving adequate data to inform these selections is an insurmountable obstacle when considering a mere 20 matches per draft phase.
Moreover, many combinations remain unexplored; constructing a team composed entirely of support characters would be an unlikely strategy. The infrequent selections of certain champions—some appearing in over 40% of matches while others may only see action once in a major tournament—could be attributed to inherent weaknesses, or perhaps innovative team strategies. When an unorthodox selection such as a quintet of support heroes occurs, evaluating that decision poses a significant challenge for analysts.
This conundrum is a persistent one in the esports data science landscape. However, I maintain that an innovative solution is viable—though, akin to previous discussions, it hinges on generating sufficient data.
By employing agents trained to perform at a professional level in League of Legends and Dota 2, we could simulate matches relentlessly, assigning random team compositions for comparison. While arduous, particularly with the need for re-training post-patch, this methodology could provide answers to pivotal questions, such as the comparative effectiveness of champions like Renekton versus Ornn.